Agentic AI: Navigating the New Era of Autonomous Decision-Making
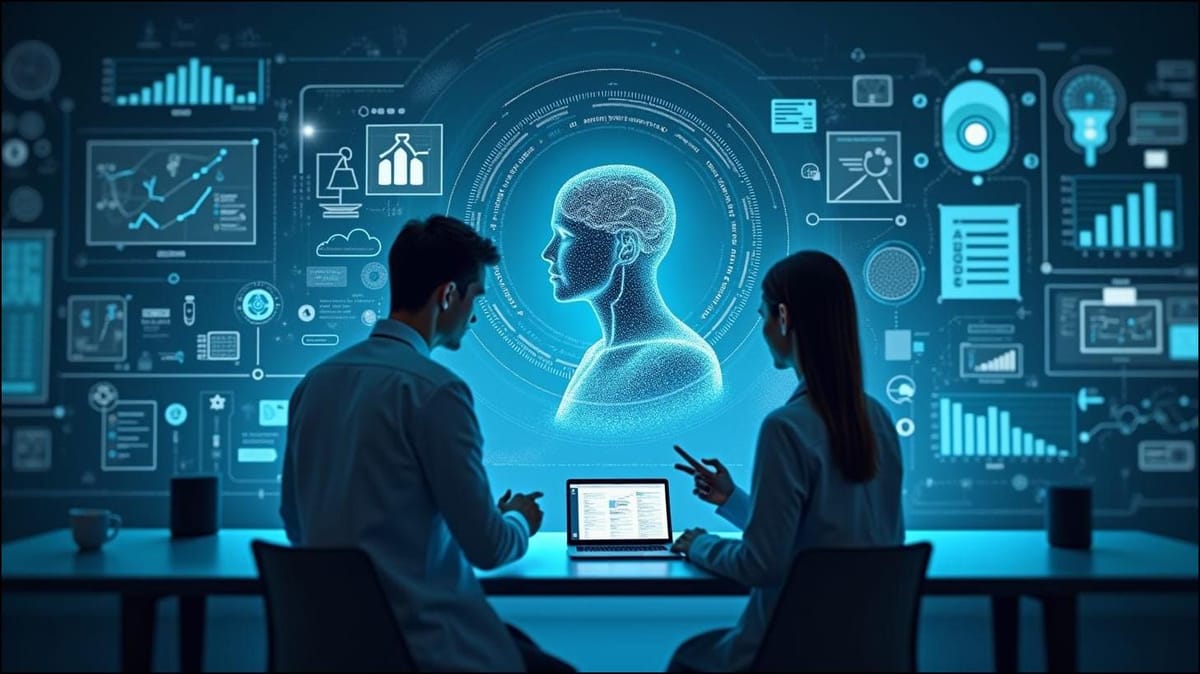
Understanding the Evolution and Impact of Agentic AI
As artificial intelligence (AI) continues to evolve, the concept of "AI's next act is agentic" marks a significant transition from mere computational roles to autonomous, action-oriented capabilities. This shift is reshaping various sectors by enabling AI systems to make independent decisions, enhancing efficiency and problem-solving potential. However, it also raises ethical and societal questions about accountability and bias. Understanding this evolution is crucial as we navigate the opportunities and challenges presented by agentic AI, setting the stage for an insightful exploration into this transformative technological development.
Understanding Agentic AI
The concept of agentic AI marks a significant evolution in the artificial intelligence domain, where systems progress from mere computational tools to entities capable of making autonomous decisions. This transformation embodies a shift towards AI systems that not only analyse data but also act upon it in real-world scenarios, effectively taking on roles traditionally occupied by humans. As these systems become more sophisticated, they navigate complexities in various environments, showcasing decision-making capabilities that adapt to changing conditions.
Advancements in machine learning algorithms and data processing have facilitated this evolution, enabling AI to operate with a degree of agency. For instance, in sectors like healthcare, AI systems are now being used to analyse patient data for diagnostics and treatment recommendations, contributing actively to decision-making processes that can significantly affect patient outcomes. Similarly, in business, AI-driven tools are managing supply chains and optimizing logistics autonomously, allowing companies to enhance operational efficiencies and reduce costs.
This agentic shift isn't just about improving efficiency; it fundamentally alters the interaction between humans and machines. As AI systems take on greater responsibilities, they challenge our understanding of autonomy and intelligence. The evolution toward agentic AI emphasizes the necessity for robust ethical frameworks and governance to ensure that these technologies act not only efficiently but also responsibly, considering the implications of their decisions in diverse contexts.
Integration of Agency in AI Systems
The integration of agency into AI systems is gaining momentum, particularly in sectors like business and healthcare. This trend is characterized by autonomous decision-making systems capable of analysing data, making predictions, and executing actions with minimal human intervention. For instance, in business, AI-driven analytics platforms are enabling organizations to identify market trends and make real-time pricing adjustments, enhancing competitiveness. Companies like Amazon utilize AI for demand forecasting, which optimizes inventory levels and reduces costs, illustrating how agency boosts operational efficiencies.
In healthcare, AI agents are transforming patient care. Predictive algorithms can now assess patient data to suggest preventative measures or indicate required interventions. Systems developed by IBM Watson Health exemplify this trend by providing evidence-based recommendations for treatment, significantly improving decision quality for healthcare professionals. These autonomous systems are reshaping industries by streamlining processes, enhancing accuracy, and reducing human error, ultimately leading to better outcomes and increased productivity.
Ethical Implications of Agentic AI
The integration of agentic AI across various industries has been revolutionary, bringing profound changes in how organizations operate. In healthcare, AI-powered systems such as chatbots and virtual health assistants have significantly enhanced patient interaction. For instance, the use of AI in managing patient inquiries through chatbots reduces response times and lowers the burden on human staff. These systems can triage patients based on symptoms, ultimately improving access to care and streamlining workflows.
In customer service, companies are leveraging AI to improve user experience. Intelligent virtual agents can handle routine inquiries, ensuring that human agents focus on more complex issues. For example, a major retail corporation reported a 40% increase in customer satisfaction after implementing an AI-driven chat support system, significantly improving response accuracy and consistency.
Supply chain management also benefits tremendously from agentic AI solutions. AI can optimize inventory management, predict demand fluctuations, and streamline logistics. A prominent logistics firm utilized AI algorithms to analyse shipping data, yielding a 20% reduction in operational costs and a notable increase in delivery efficiency. These improvements underscore how agentic AI is not just enhancing processes but driving significant operational transformations across industries.
Technological Innovations Driving Agency
Technological innovations such as natural language processing (NLP), reinforcement learning, robotics, and the Internet of Things (IoT) are significantly enhancing agentic AI. Advances in NLP, like transformers and large pre-trained models, have democratized access to sophisticated text and speech comprehension, enabling machines to interact more effectively with humans. Reinforcement learning, particularly in gaming and autonomous systems, allows AI agents to learn optimal behaviours by taking actions in complex environments and receiving feedback, which is pivotal for decision-making processes.
In robotics, recent breakthroughs in sensory technology and AI algorithms are enabling robots to perform versatile tasks with greater autonomy, from manufacturing to healthcare. Furthermore, IoT plays a critical role by connecting devices and sensors to streamline operations and data collection, resulting in smarter systems that can self-regulate and optimize functions. Together, these technological advancements are harmonizing to foster the evolution of intelligent agents, enabling them to act with a higher degree of agency and versatility.
Challenges and Risks Involved
The integration of agency into AI systems presents numerous challenges and risks that could significantly affect their adoption and advancement. One of the primary concerns is technical limitations. Agentic AI often relies on complex algorithms and vast amounts of data; this dependency can lead to difficulties in accuracy and reliability, particularly in dynamic environments where adaptability is crucial. If AI systems misinterpret data or fail to evolve with changing conditions, they may make poor decisions, resulting in potentially harmful consequences.
Public perception also plays a critical role in the acceptance of agentic AI. Mistrust and scepticism about decision-making processes conducted by machines can hinder widespread adoption. Many consumers fear that AI systems could replace human jobs, make biased decisions, or be vulnerable to misuse. Additionally, security concerns regarding data privacy and susceptibility to cyber threats are paramount; agentic AI systems could be targets for hostile entities looking to exploit weaknesses. Such vulnerabilities can lead to significant socio-economic ramifications, necessitating robust measures to mitigate risks.
Together, these factors create an environment where the development of agentic AI technologies can proceed with caution, influencing regulatory frameworks and innovation pathways while requiring transparency to foster trust among users and stakeholders.
Future Perspectives and Recommendations
As AI continues to evolve, the integration of agency will likely manifest through enhanced autonomous decision-making capabilities across various sectors. Anticipated advancements may involve greater self-learning algorithms, allowing systems to adapt dynamically to complex environments. This reflects a shift towards more sophisticated agentic behaviours where AI not only assists but also initiates actions based on predictive analytics. For instance, in healthcare, AI systems could autonomously manage treatment plans by analyzing real-time patient data, thus potentially increasing efficiencies and saving lives. However, this growing autonomy necessitates a robust ethical framework to mitigate risks associated with biased decision-making or lack of accountability.
For businesses and policymakers, it is crucial to establish comprehensive regulations that ensure ethical standards in AI agency. Stakeholders should prioritize transparency, mandating AI systems to explain their decision-making processes to bolster trust. Investment in interdisciplinary research on the societal impacts of AI is also recommended to anticipate potential repercussions. Additionally, collaborative frameworks involving technologists, ethicists, and the community can help navigate the complexities of deploying agentic AI. By fostering a proactive approach, we can harness the full potential of AI while mitigating its risks effectively.
Conclusions
The transition to agentic AI represents a pivotal evolution in the field of artificial intelligence, moving from theoretical computing to practical, decision-making tools that can profoundly impact industries like healthcare, customer service, and supply chains. While this shift promises enhanced efficiencies, it brings ethical considerations and technical challenges that must be prudently managed. As AI continues to develop and integrate more autonomously into our daily lives, stakeholders must champion responsible use and robust ethical frameworks to ensure equitable and beneficial outcomes for society.